top of page

Lab. LGI2P
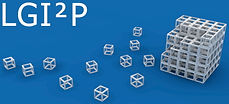
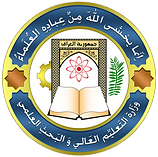

وزار Ø© التعليم العالي ÙˆØ§Ù„Ø¨ØØ« العلمي - العراق
الجامعة التقنية الشمالية
الدكتور ØØ³Ù† كريم عبد الرØÙ…Ù†
اختصاص معالجة الصور الرقمية وامنية المعلومات
Corner and Junction Detection Method
In digital images, corners and junctions get affluent information like delimitation of objects, they constitute important landmarks.
Many algorithms for detecting the corners have been developed up to now. Indeed, corner detection is often an important step in various image-understanding and scene-reconstructing systems.
The corner detectors should satisfy the following criteria:
-
All the true corners and no false corners should be detected.
​
-
The corners should be located precisely.
​
-
The detectors should not be sensitive to noise in images. very point.
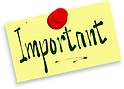

PROPOSED METHOD EXPERIMENTS
we proposed new robust corner detection method and our experiments carried out on synthetic images and real image to compare corner detectors. Concerning the synthetic images, the signal is corrupted by a Gaussian noise over different Signal-to-Noise Ratios (SNR). Then, the Root-Mean-Square Error (RMSE) is computed between the 31 true corners and the 31 ”best” corners extracted by the corner detectors. Our proposed approach remains more stable and robust to noise than the nine compared methods.
Our method was implemented and tested. The following was found out:
-
The detection of corners is highly reliable.
​
-
The localization errors are small.
​
-
The sensitivity to noise is low.
PROPOSED METHOD RESULTS WITH = 1

Experiments results with synthetic image

- Our proposed method remains more stable and robust to noise than the nine compared methods. I published the two close results to our method results.
PROPOSED METHOD
Aach method [1]
True corner = + , False corner = +, Forget corner = +.
Shui method [2]
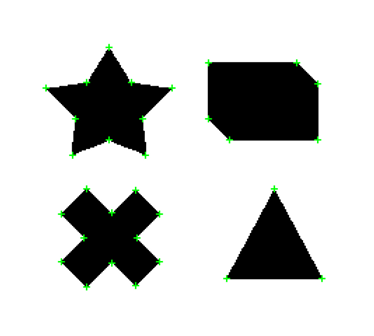
SNR= , RSME=0.000

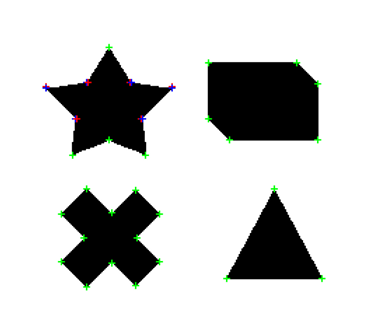
SNR= , RSME=0.4399

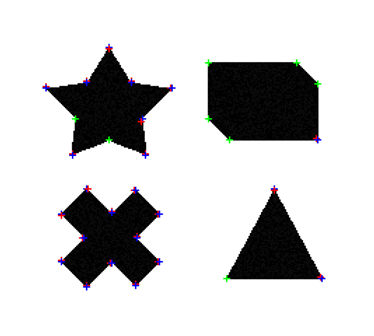
SNR= , RSME=0.9672

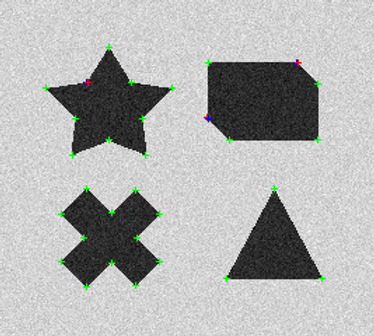
SNR=11 dB, RSME=0.3113
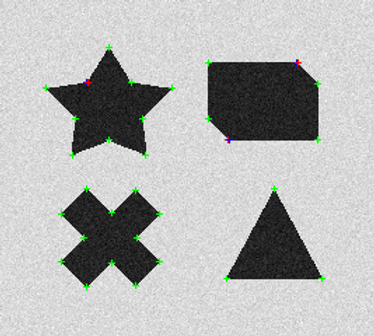
SNR=10 dB, RSME=0.3592
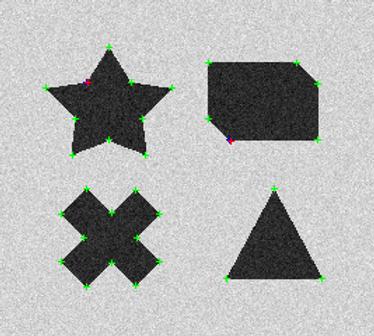
SNR= 9 dB, RSME=0.3111

SNR=11 dB, RSME=0.4751
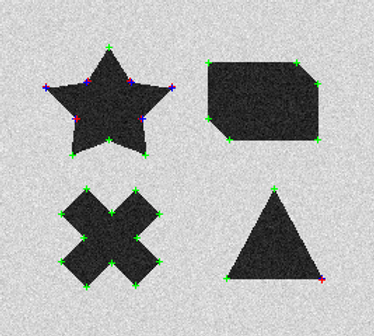
SNR=10 dB, RSME=0.5388
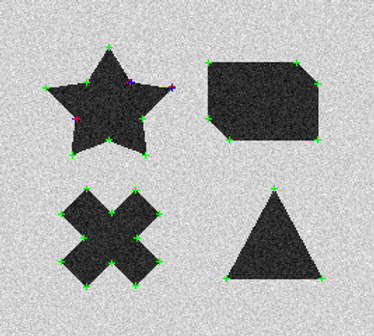
SNR= 9 dB, RSME=0.3119

SNR=11 dB, RSME=0.9672
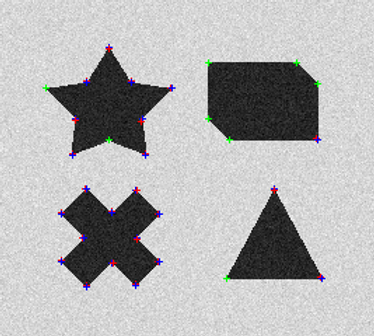
SNR=10 dB, RSME=0.9673

SNR= 9 dB, RSME=0.9158
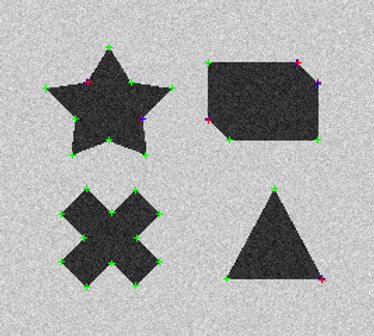
SNR= 8 dB, RSME=0.4399

SNR= 7 dB, RSME=0.4016
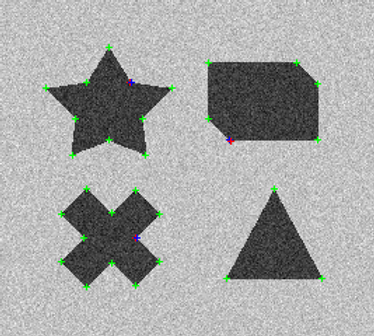
SNR= 6 dB, RSME=0.3593
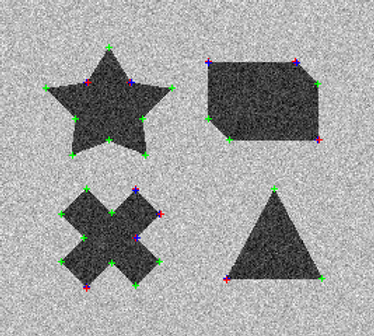
SNR= 5 dB, RSME=0.5957

SNR= 4 dB, RSME=1.8050
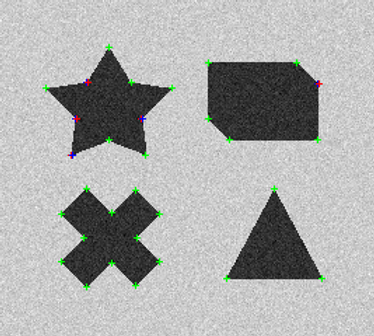
SNR= 8 dB, RSME=0.4016

SNR= 7 dB, RSME=0.6476
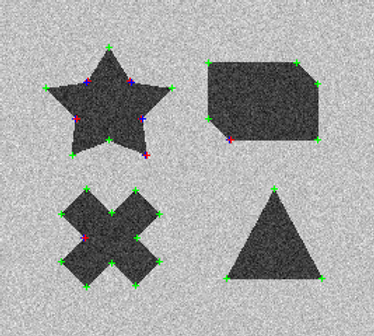
SNR= 6 dB, RSME=0.5388
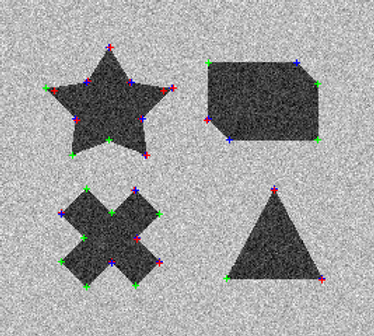
SNR= 5 dB, RSME=4.0521
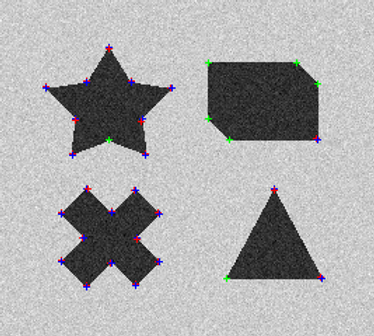
SNR= 8 dB, RSME=1.0000

SNR= 7 dB, RSME=1.9610
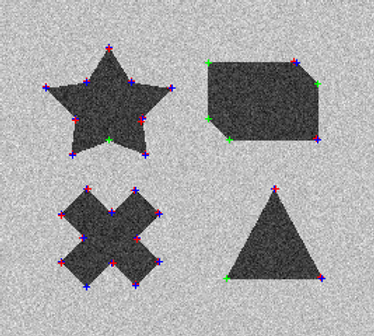
SNR= 6 dB, RSME=3.3118
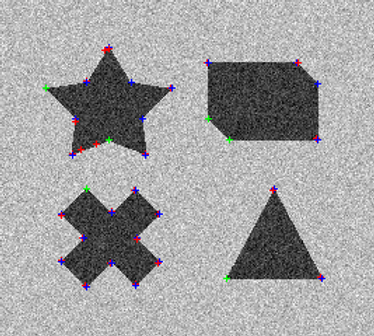
SNR= 5 dB, RSME=5.7712

SNR= 4 dB, RSME=5.8101
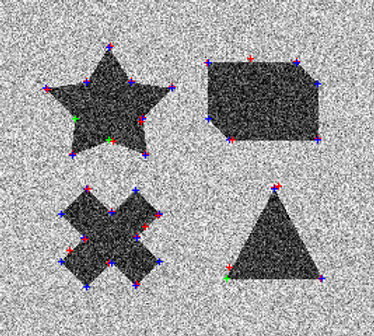
SNR= 4 dB, RSME=8.5647
Experiments results with real image
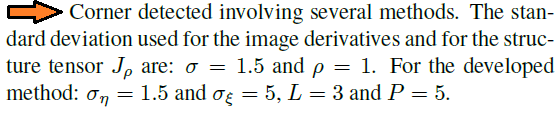
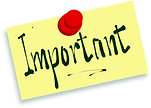
To make the comparison easier, only 180 best interest points are extracted for each method
PROPOSED METHOD
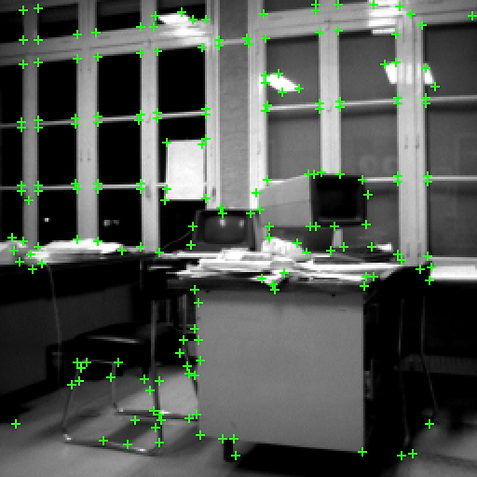
Kitchen method[3]
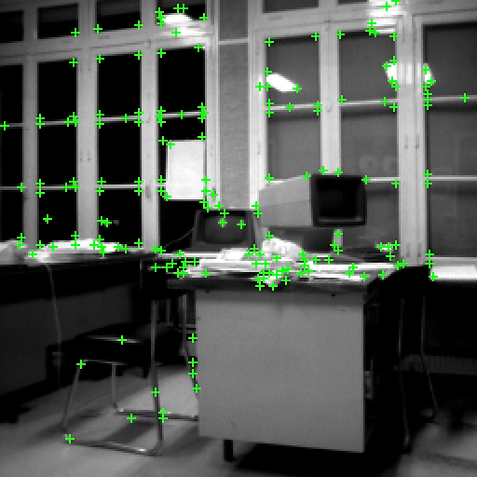
Fast method [6]
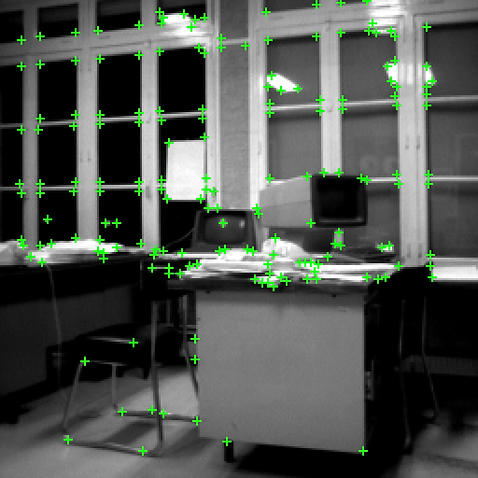
Aach method [1]
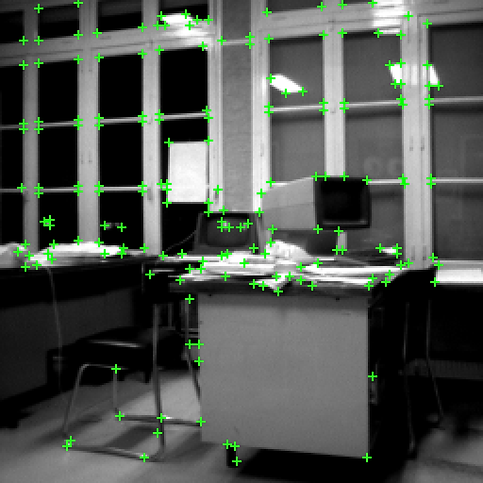
Shi-Tomasi method[4]
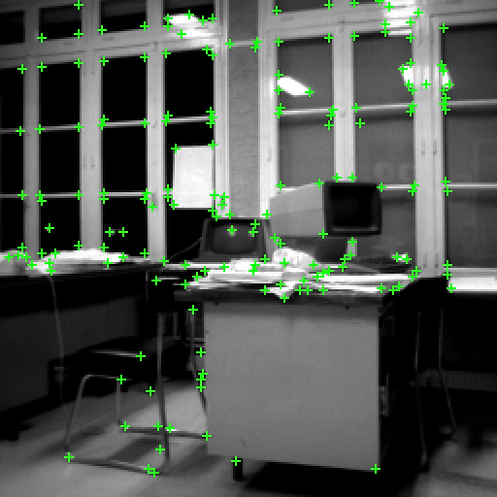
Forstner method [7]
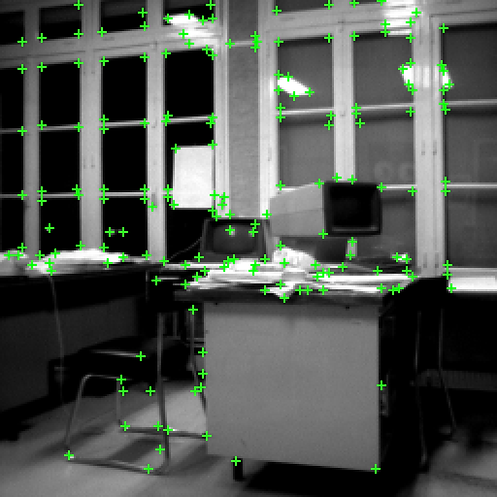
Shui method [2]
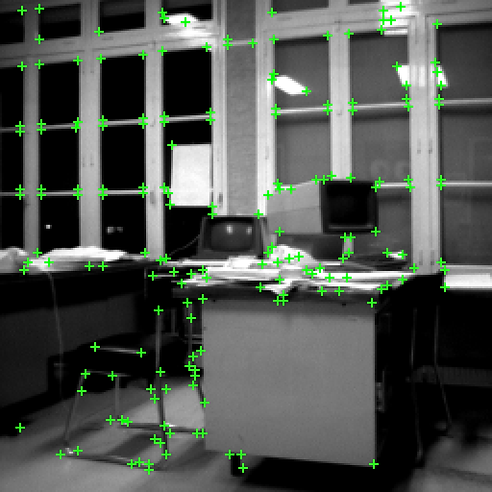
Xia method[5]
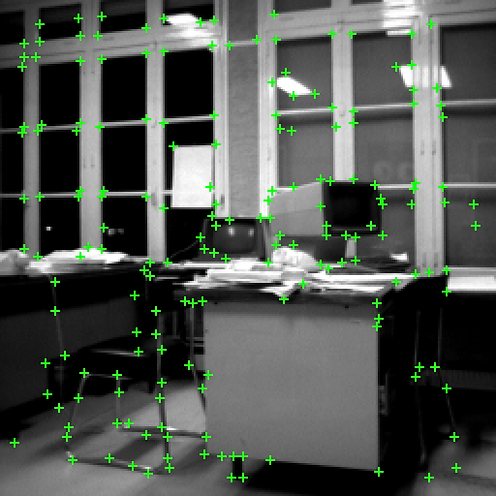
Harris method [8]

Koethe method [9]
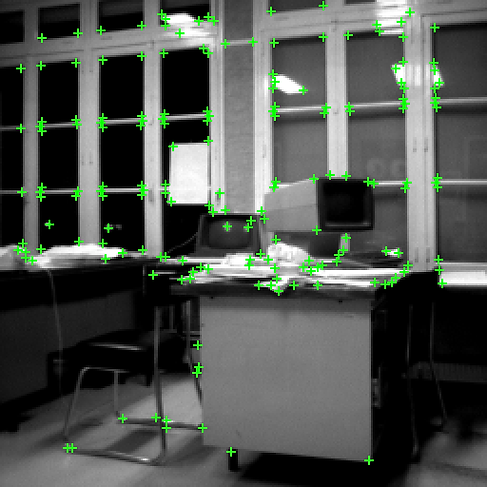
References
[1] T. Aach, C. Mota, I. Stuke, M. Muhlich, and E. Barth,“Analysis of superim oriented patterns,” IEEETIP, vol. 15, no. 12, pp. 3690–3700, 2006.
[2] P.-L. Shui and W.-C. Zhang, “Corner detection and classification using anisotropic directional derivative representations,” IEEE TIP, vol. 22, no. 8, pp. 3204–3218, 2013.
[3] L. Kitchen and A. Rosenfeld, “Gray-level corner detection,”
Tech. Rep., DTIC Document, 1980.
[4] J. Shi and C. Tomasi, “Good features to track,” in IEEE CVPR, 1994, pp. 593–600.
[5] G.S. Xia, J. Delon, and Y. Gousseau, “Accurate junction detection and characterization in natural images,” IJCV, vol. 106, no. 1, pp. 31–56, Springer, 2014.
[6]E. Rosten, R. Porter, and T. Drummond, “Faster and better: A machine learning approach to corner detection,”IEEE TPAMI, vol. 32, no. 1, pp. 105–119, 2010.
[7] W. Forstner, “A feature based correspondence algorithm for image matching,” International Archives of Photogrammetry and Remote Sensing, vol. 26, no. 3, pp. 150–166, 1986.
[8] C. Harris and M. Stephens, “A combined corner and edge detector.,” in Alvey Vision Conference, Citeseer, vol. 15, p. 50, 1988.
[9] U. K¨othe, “Edge and junction detection with an improved structure tensor,” in Joint Patt. Rec. Symp.,
Springer, pp. 25–32, 2003.
​
​
​
​
​
​
​
​
​
​
​
There are more experiments I will publish in the website later.
​
bottom of page